Hi, I'm Rimita Lahiri.
A
I am a PhD researcher. My research focuses on understanding human behaviour from multimodal behavioral signals in real-world settings.
About
I am a doctoral candidate in the Ming Hsieh Department of Electrical and Computer Engineering at University of Southern California, Viterbi School of Engineering. I am a member of the Signal Analysis and Intepretation Laboratory and currently advised by Prof. Shrikanth Narayanan. Prior to USC, I worked as a Researcher at TCS Innovation Labs, Kolkata from 2016 to 2018. I received my Masters in 2016 from Jadavpur University where I worked under supervision of Prof. Amit Konar. I received my Bachelors in Electronics and Communication Engineering from Heritage Institute of Technology Kolkata in 2014.
My research interests broadly span across domains of multimodal machine learning, behavior signal processing and applications of speech and language in clinical domain. I am specifically interested in developing machine learning and deep learning based solutions that help us to understand and analyze human behavioral traits in real-world settings.
Experience
- Leading and coordinating research and collaboration efforts of CARE project with 7 PhD students
- Developing novel and efficient algorithms for speech and language processing tasks using machine learning for child speech understanding specifically in te domain of autism research
- Developing contextual measures for understanding children behaviour using multimodal signals
- Mentored in exploreCSR outreach workshop
- Significantly contributing to grant proposals
- Developed an ASR solution for overlapped multi-talker use case and analyzed the impact of leveraging speaker embeddings for the same.
- Developed and analyzed the impact of adding synthetically generated data on the performance of intent/slot filling models using state of the art paraphrasing networks.
- Developed a meta-learning based ASR framework for multilingual use case with enhanced performance.
News
Projects
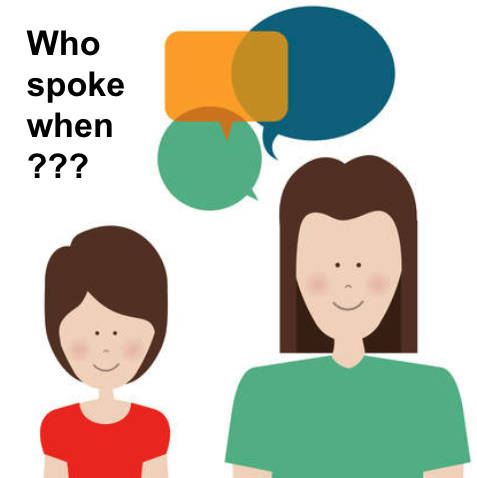
Exploration of adversarial learning strategies for speaker classification in child-inclusive interactions.
- Computational approach for speaker classification in semi-naturalistic interactions between child and adult.
- Supervised binary classification setup for speaker label prediction.
- Framework is trained to address 2 main sources of variability: age of the child, data source collection location.
- Adversarial learning using 2 methods : inverted label loss and gradient reversal layer.
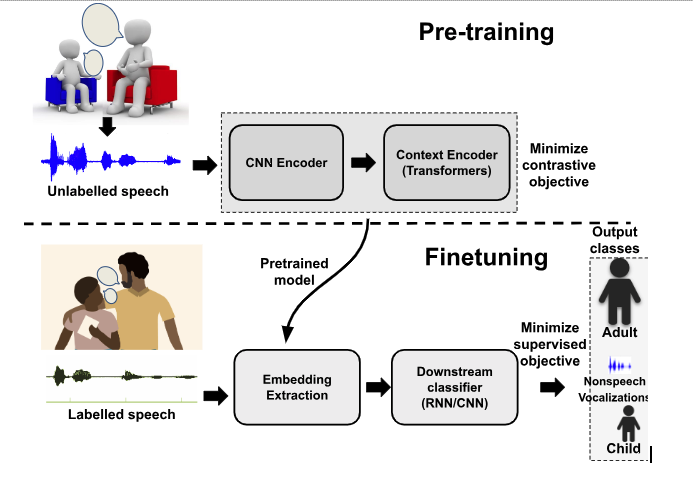
Exploration of self-supervised learning strategies for speaker classification in child-inclusive interactions.
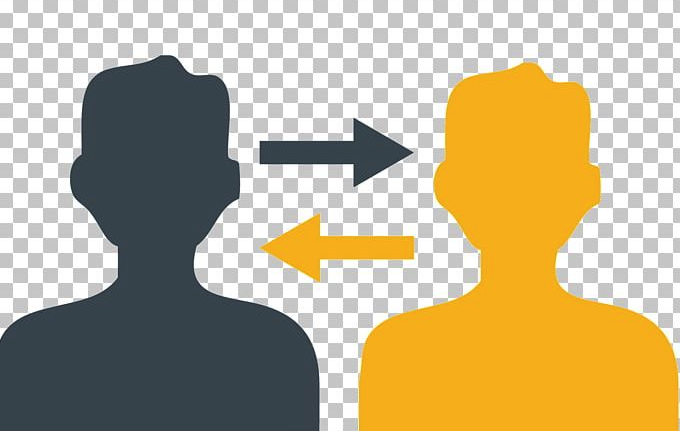
Exploration of knowledge-sriven statistical approaches for modeling intepersonal synchrony in clinical interactions involving children
- Introduction of 3 different objective measures of interpersonal synchrony across vocal and lexical modalities.
- For vocal prosodic and spectral features Dynamic Time Warping Distance(DTWD), Squared Cosine Distance of (featurewise) Complexity(SCDC) are used and for lexical features Word Movers' Distance(WMD) is used.
- The complementarity of these synchrony measures was studied by an ad-hoc classification experiment to distinguish between children with and without autism.
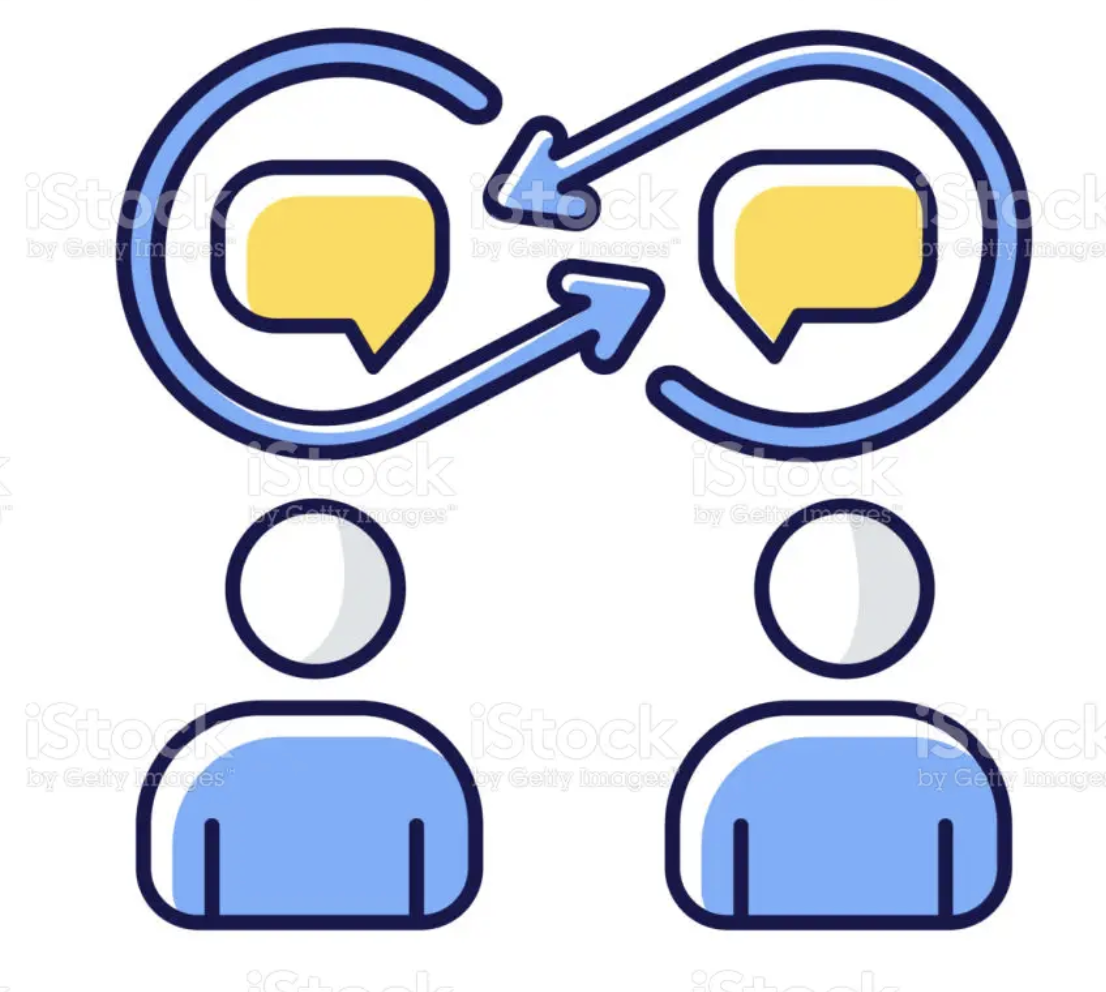
A context-aware computational approach for quantification of entrainment in dyadic interactions.
- We use conformers to capture both local and global context.
- We use cross-attention to jointly modeling both the interlocutors in a dyadic interactions
- Experimental results show evidence of statistically significant association between the introduced measure and clinically meaningful behavioral codes.
Skills
Scripting Languages
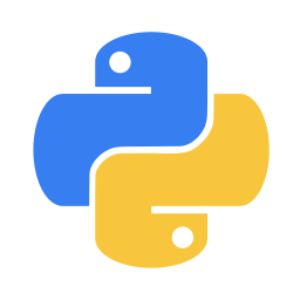
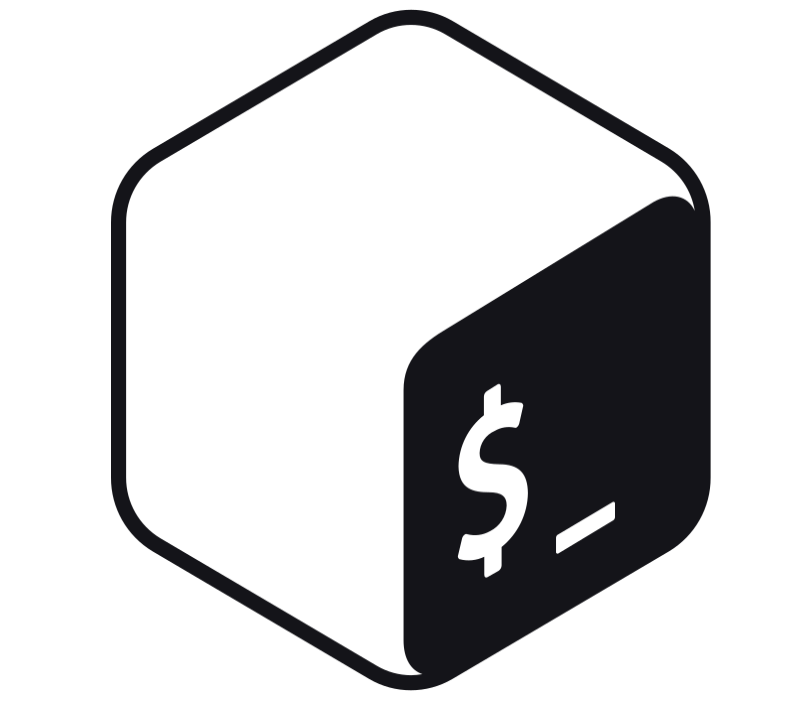
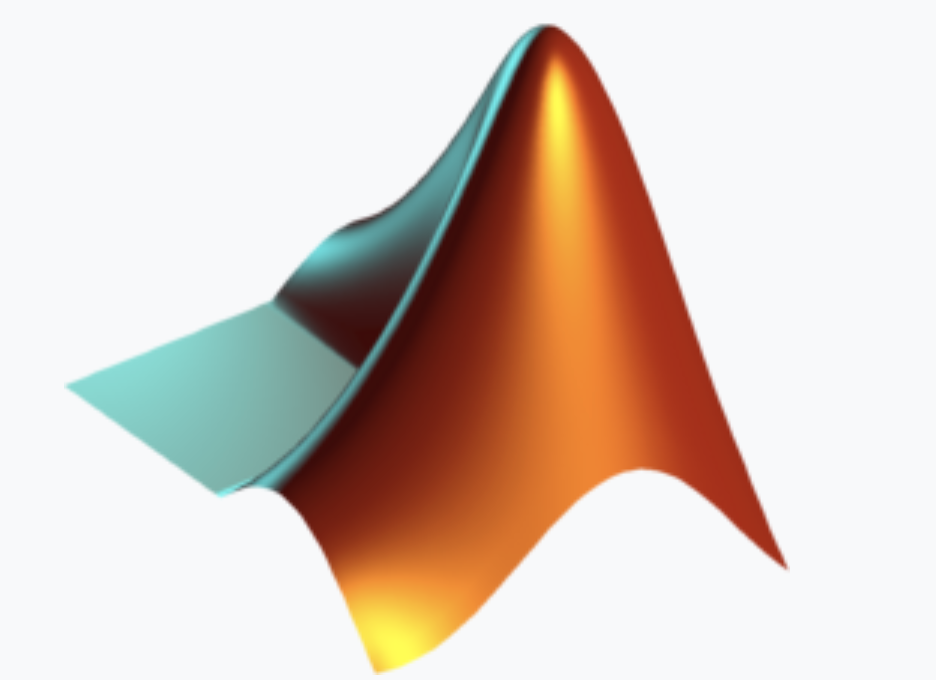
Tools/Frameworks
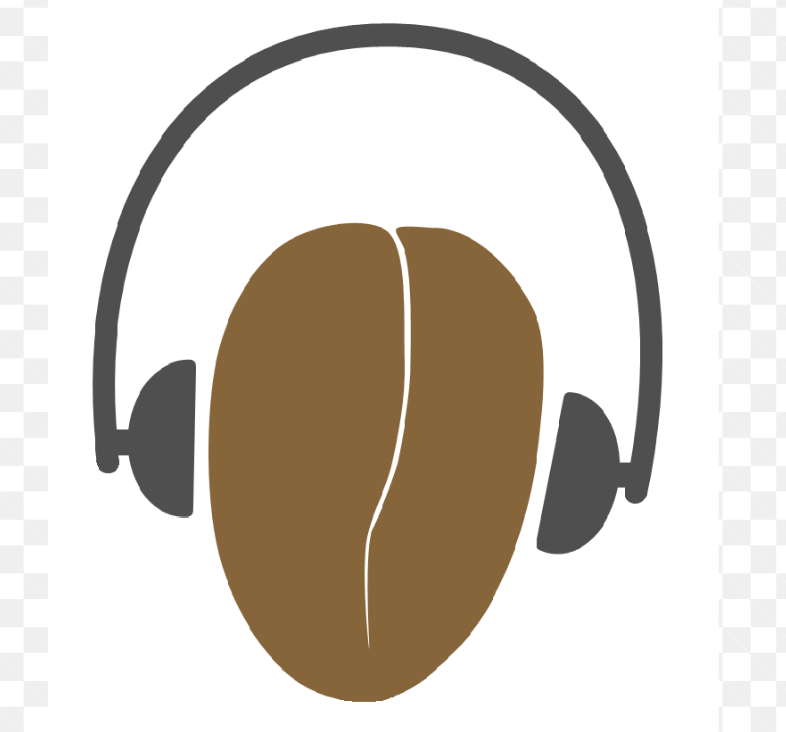
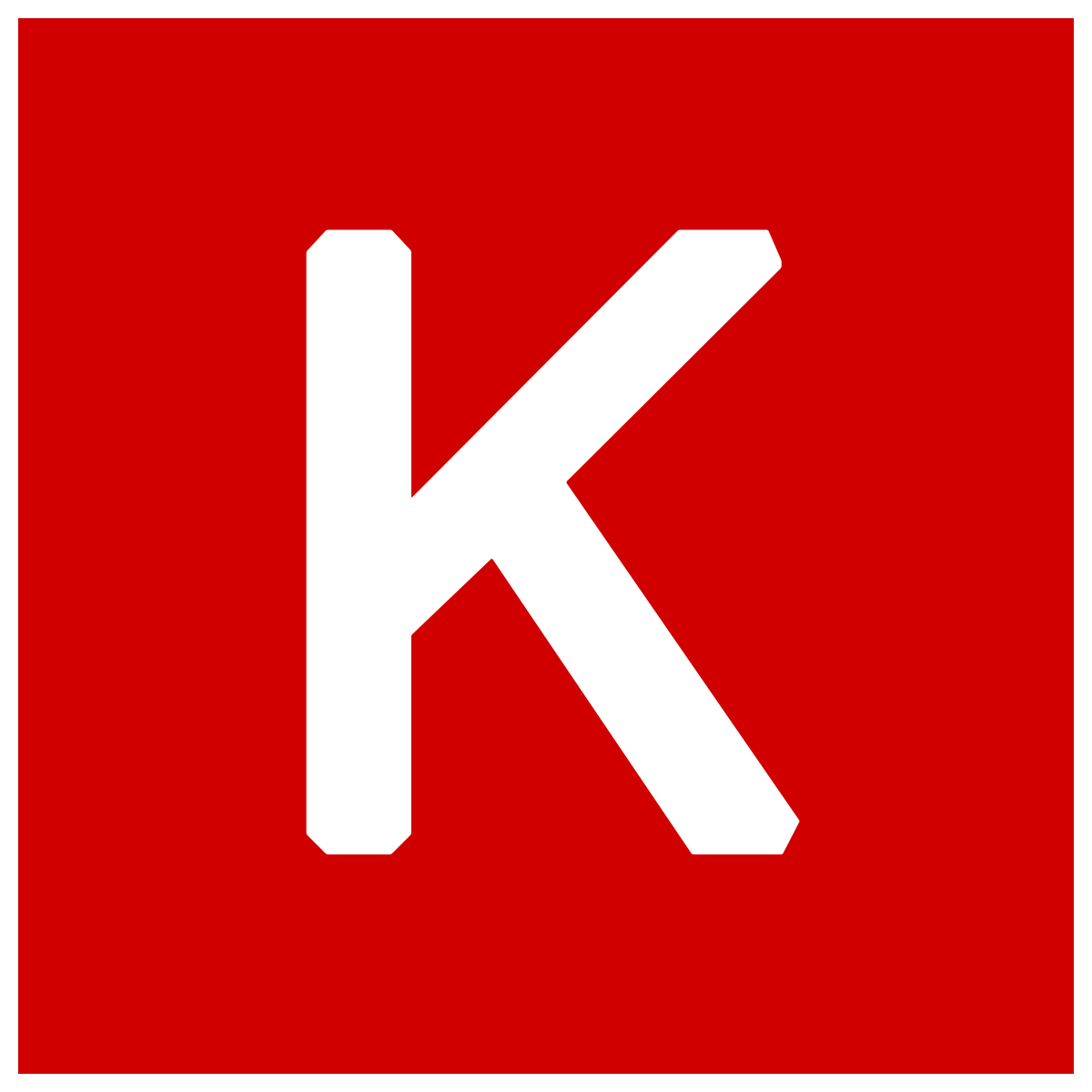
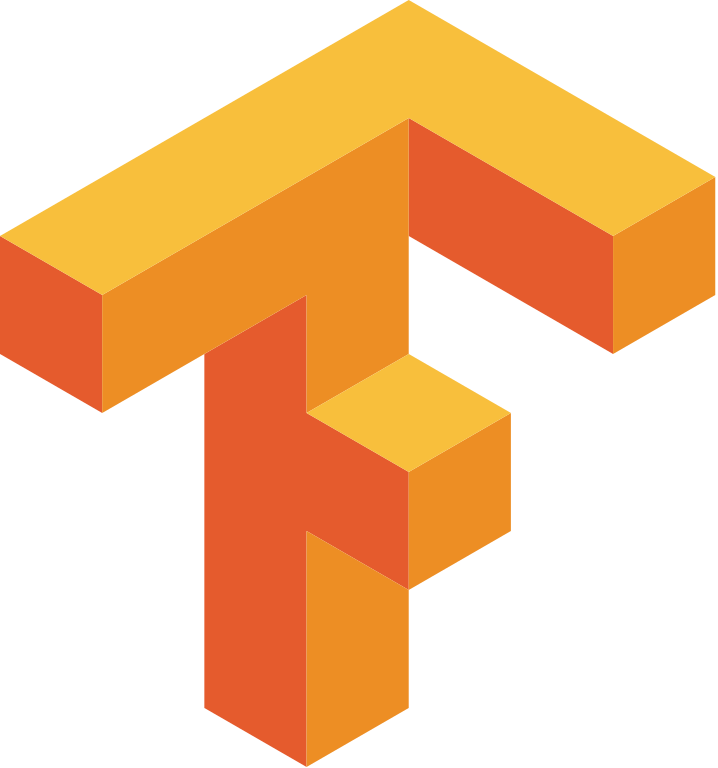
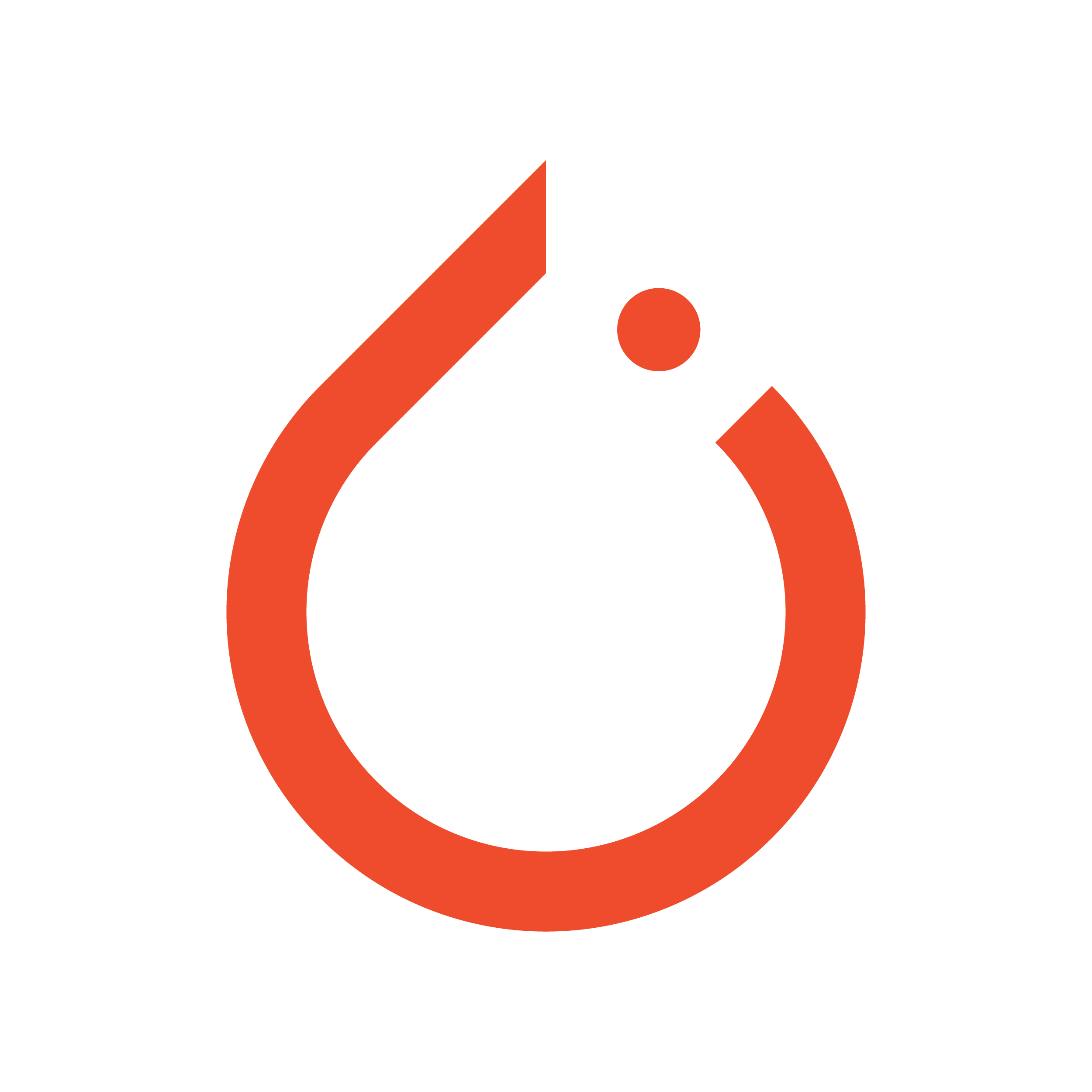
Education
University of Southern California
Los Angeles, USA
2018 - Present
Degree: PhD
Department: Ming Hsieh Department of Electrical & Computer Engineering
CGPA: 3.70/4.0
- Machine Learning
- Applied Natural Language Processing
- Foundations of Algorithms
- Affective Computing
Relevant Courseworks:
Kolkata, India
2014-2016
Degree: Master of Engineering
Department: Electronics and Telecommunication Engineering
CGPA: 9.94/10.0
- Artificial Intelligence and Soft Computing
- Digital Signal Processing
- Robotics and Computer Vision
- Computational Biology and Bioinformatics
Relevant Courseworks:
Heritage Institute of Technology, Kolkata
Kolkata, India
2010-2014
Degree: Bachelor of Technology
Department: Electronics and Communication Engineering
CGPA: 8.92/10.0